A computer technique based on machine learning (ML) has been created by researchers at the Indian Institute of Technology (IIT) Madras to improve the identification of cancer-causing tumours in the brain and spinal cord.
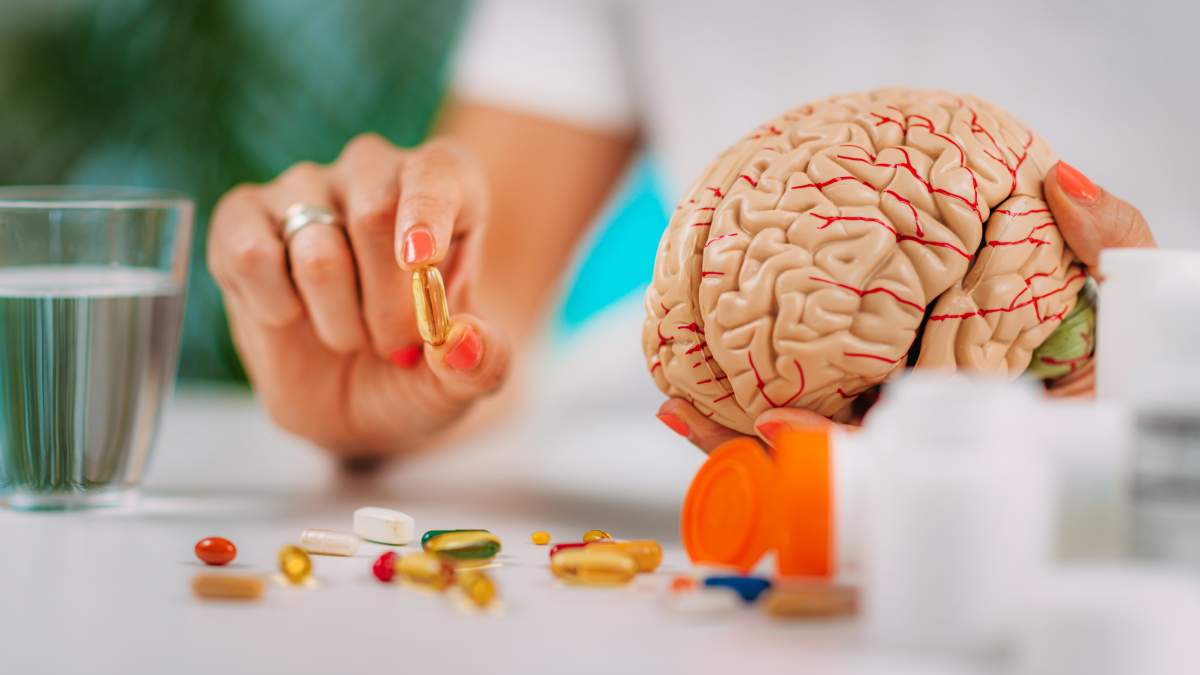
Know About the Tumour
Glioblastoma is a rapidly and aggressively developing cancer of the brain and spinal cord. Although research has been done to understand this tumour, the therapeutic options are still limited, and the expected survival time after diagnosis is less than two years.
The Great Creation
To specifically detect driver mutations and passenger mutations in glioblastoma, the technology known as aGBMDriver (GlioBlastoma Mutiforme Drivers) was created. The tool is accessible to everyone online. The GBMDriver website can be reached at: https://web.iitm.ac.in/bioinfo2/GBMDriver/index.html. Driver mutations are those that cause cell proliferation and tumour formation, whereas passenger or ‘hitchhiker’ mutations, which account for around 97% of all carcinogenic mutations, do not.
Researchers Say
According to a statement from Prof. M. Michael Gromiha of the Department of Biotechnology at IIT Madras, “We have identified the important amino acid features for identifying cancer-causing mutations and achieved the highest accuracy for distinguishing between driver and neutral mutations.” In order to build drug design strategies, Gromiha continued, “We hope that this tool (GBMDriver) could help to prioritise driver mutations in glioblastoma and assist in identifying potential therapeutic targets.”
The scientists examined 8,728 passenger mutations and 9,386 driver mutations in glioblastoma in order to create this web server. In a blind set of 1809 mutants, driver mutations in glioblastoma were detected with an accuracy of 81.99%, which is superior to current computational techniques. The protein sequence is the sole determinant in this technique.
Benefits and Advantages
The peer-reviewed journal Briefings in Bioinformatics published their findings. Other diseases can also benefit from using the ML tool. The technique might be one of the crucial factors in determining a disease’s prognosis. It is a useful tool for locating pharmacological targets that are specific to a mutation while creating treatment plans. According to Medha Pandey, a PhD student at IIT Madras, “We anticipate that the method is helpful to prioritise driver mutations in glioblastoma and assist in identifying therapeutic targets.”